We are excited to present you with Part 4 of our in-depth analysis of the utilization of artificial intelligence (AI) in the financial sector, focusing on bridging the insights gained from the FFIEC’s Request for Information (RFI) with the findings of the analysis performed by the Firm. In this part, we focus on Cybersecurity risk related to AI in financial institutions (Question 7).
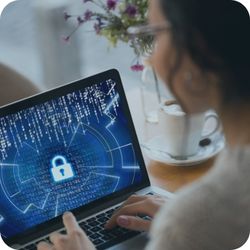
Cybersecurity concerns related to AI were noted by 31% of the organizations. This lower percentage might indicate a belief that:
- The specific risks AI poses still need to be fully understood and experienced (most likely).
- Potential need for awareness or readiness (likely).
- Existing cybersecurity measures are adequate (less likely).
The practices that manage these risks vary, highlighting the complexity and evolving nature of AI-related cybersecurity threats. The identified barriers and challenges suggest that much work still needs to be done to design and implement adequate security controls specific to AI. This lower percentage underscores the need for ongoing research, collaboration, and knowledge sharing among financial institutions, cybersecurity experts, and AI developers to ensure AI’s secure use and implementation in the financial sector.
For previous analysis, refer to:
- Strategic Inquiry into AI Adoption: Federal Agencies Seek Stakeholder Insights on AI in Financial Institutions – Part 1 – Mijares Consulting
- Strategic Inquiry into AI Adoption: Federal Agencies Seek Stakeholder Insights on AI in Financial Institutions – Part 2 – Mijares Consulting
- Strategic Inquiry into AI Adoption: Federal Agencies Seek Stakeholder Insights on AI in Financial Institutions – Part 3 (A): Community Institutions – Mijares Consulting
- Strategic Inquiry into AI Adoption: Federal Agencies Seek Stakeholder Insights on AI in Financial Institutions – Part 3 (B): Community Institutions – Mijares Consulting
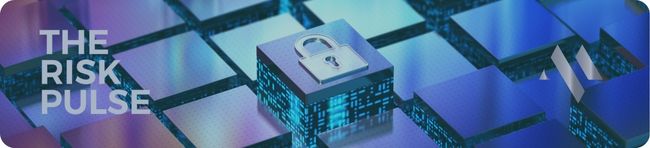
Executive Summary
Financial institutions progressively integrate AI and ML technologies into their operations while maintaining a vigilant posture on cybersecurity risks. Although no significant AI-related incidents in financial institutions have been directly observed since February 2025, potential vulnerabilities such as data poisoning, adversarial manipulations, and third-party exposures remain substantial. Organizations are implementing strong risk management frameworks to mitigate these risks, including data protection measures, continuous monitoring, and security-by-design principles. Key insights from the FFIEC AI RFI and World Economic Forum reports emphasize the importance of a comprehensive, multi-layered approach encompassing stringent access controls, lifecycle risk management, and proactive governance to safeguard sensitive information and maintain algorithmic integrity.
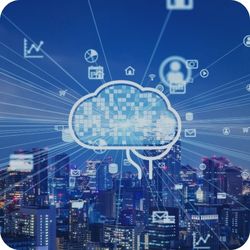
Despite these advances, challenges persist due to AI models’ inherent complexity and opacity, which complicate vulnerability detection and risk mitigation. The rapid pace of technological evolution and a shortage of specialized expertise further intensify these challenges, necessitating ongoing investment in workforce development and cross-disciplinary collaboration. In this dynamic threat environment, tailored cybersecurity controls and proactive incident response strategies are critical to balancing innovation with effective risk management. By embedding these practices into their operational frameworks, financial institutions can sustain trust, enhance resilience, and ensure the secure deployment of AI technologies.
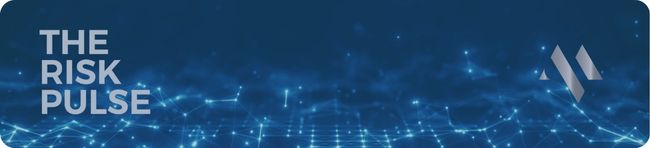
Question 7: Have financial institutions identified particular cybersecurity risks or experienced such incidents with respect to AI?
If so, what practices are financial institutions using to manage cybersecurity risks related to AI? Please describe any barriers or challenges to the use of AI associated with cybersecurity risks. Are there specific information security or cybersecurity controls that can be applied to AI?
Cyber security risks or incidents with respect to AI
Cyber security risks or incidents with respect to AI As of February 2025, no major cybersecurity incidents have been directly linked to AI/ML models in financial institutions. These technologies primarily enhance operational efficiency, client service, and risk management.
However, the financial sector remains cautious about potential vulnerabilities related to data and algorithms.
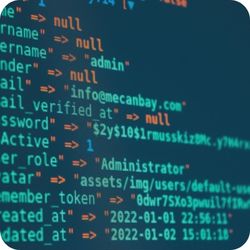
Data-related risks, such as poisoning training datasets or adversarial manipulations, can disrupt fraud detection and credit risk systems. Sensitive training data risks leakage, theft, or misuse, leading to regulatory penalties and customer trust erosion. Algorithmic vulnerabilities, often present in open-source frameworks, can result in significant errors under adversarial conditions or unfamiliar scenarios, impacting critical banking functions.
Reliance on third-party vendors, including fintech firms, adds another layer of exposure, necessitating more effective oversight. Despite technological advancements, human error, such as phishing, remains a prominent threat, underscoring the need for continuous employee training.
Financial institutions are implementing strong data protection, AI governance frameworks, regular security testing, and stricter third-party risk management to mitigate these risks. While no significant incidents have occurred, the industry remains alert, proactively addressing the evolving risks of AI technologies to safeguard trust and operations.
What practices are financial institutions using to manage cybersecurity risks related to AI?
Financial institutions adopt a comprehensive, multi-layered approach to effectively manage cybersecurity risks related to AI. This approach includes strong security measures, stringent data protection practices, and risk management frameworks. They enforce access controls, infrastructure protection, incident response, and regulatory compliance with frameworks like FFIEC and NIST. This proactive strategy ensures the safety and integrity of AI systems and the sensitive data they process.
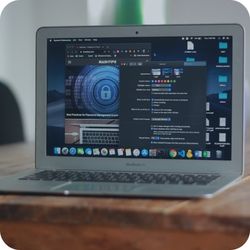
Setting clear boundaries around AI frameworks is crucial to prevent AI systems from considering unanticipated combinations of exotic factors. AI systems may rely on traditional, easily verifiable limits such as bureau scores and loan-to-value ratios to mitigate the risk of adversarial exploitation. Identifying and validating appropriate AI use cases, ensuring high-quality data, developing effective bias reduction techniques, and maintaining a precise inventory of clean training data are essential to managing AI-related risks.
Third-party risks are mitigated through vendor evaluations, audits, user access, and encrypted data management. Governance frameworks ensure algorithmic transparency and accountability through policies, audits, and AI model inventories.
Lifecycle risk management includes tracking model and data changes and continuous monitoring for model drift (the gradual degradation of a machine learning model’s performance over time), anomalies, or adversarial threats. By adopting these practices, financial institutions safeguard sensitive data, maintain AI system integrity, and enable secure, efficient AI adoption.
Barriers or challenges to the use of AI associated with cybersecurity risks
A key issue is the complexity and lack of transparency in AI models, which make identifying vulnerabilities and implementing security measures difficult. This complexity requires specialized expertise to mitigate potential threats effectively. Ensuring data quality and integrity is another critical challenge, as compromised or biased data can lead to unreliable outputs. The rapid evolution of AI further complicates the consistent application of security protocols, requiring robust data governance.
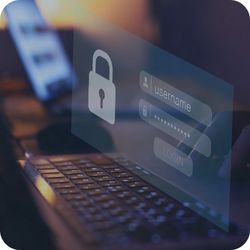
The shortage of skilled professionals in both AI and cybersecurity exacerbates these challenges, leaving institutions struggling to secure their systems. Adversarial AI techniques, such as data poisoning and model evasion, introduce sophisticated threats that require continuous monitoring and advanced countermeasures. Additionally, the need for tailored security protocols adds to resource burdens, particularly for smaller institutions, slowing innovation and market entry.
Regulatory challenges arise as AI outpaces existing frameworks, creating gaps and inconsistencies that hinder effective risk management. Human error remains a persistent issue, as phishing and data mishandling can compromise even advanced systems. Ongoing training and awareness programs are essential to address this vulnerability.
In the last four years, coordinated approaches between institutions and regulators have increased to improve effective responses to emerging threats (e.g., FFIEC AI RFI in 2021, Treasury Department AI RFI in 2024, and OCC AI research RFI in 2024). Addressing these barriers requires comprehensive risk
management frameworks, investment in workforce development, and stronger public-private collaboration to ensure secure and responsible AI use in financial services.
Are there specific information security or cybersecurity controls that can be applied to AI?
Institutions must implement comprehensive cybersecurity controls to secure AI systems in financial services, including data protection, model monitoring, user access controls, change management model monitoring, advanced monitoring, security-by-design practices, regulatory compliance, and collaboration.

Data Protection: Encryption, secure storage, and access management ensure data security at all stages, forming a strong foundation against breaches.

Monitoring and Advanced Techniques: Continuous monitoring of data and models, regular penetration tests, and techniques like differential privacy and federated learning safeguard AI systems from adversarial attacks and vulnerabilities.

Security-by-Design: Integrating security into AI development through source code reviews, SDLC including change management, vulnerability assessments, and strict model training logs prevent risks from emerging during development.

Regulatory Compliance: Adhering to standards like the Gramm-Leach-Bliley Act and ensuring vendor compliance with regulations protect data integrity and fairness. Regular audits enhance accountability.

AI-Specific Controls: Maintaining inventories of AI models, model versioning controls, auditing changes, using “golden datasets,” and monitoring insider threats improve model reliability and security.

Collaboration: Partnerships like MITRE’s ATLAS foster knowledge-sharing to address evolving threats.

Employee Training: Regular training minimizes human error, a significant cybersecurity risk, while awareness programs ensure vigilance against threats.

IT Resiliency: Disaster recovery and business continuity plans enable quick recovery from incidents, minimizing operational disruptions.
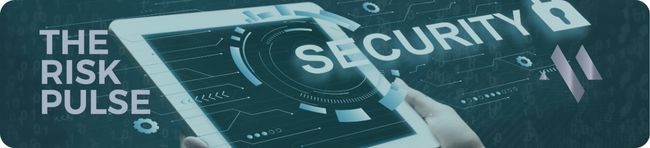
U.S. Department of the Treasury report
Managing AI-specific cybersecurity risks
In response to the Executive Order on the Safe, Secure, and Trustworthy Development and Use of Artificial Intelligence (October 2023), the U.S. Department of the Treasury released the report “Managing Artificial
Intelligence-Specific Cybersecurity Risks in the Financial Services Sector” in March 2024 (Managing Artificial Intelligence-Specific Cybersecurity Risks in the Financial Services Sector). This report highlights critical areas where financial institutions must adapt to the unique risks posed by AI technologies, especially generative AI, while seizing opportunities to enhance cybersecurity frameworks.
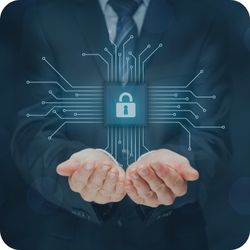
Traditional models often fail to address AI-specific risks, such as data poisoning and integrity attacks, necessitating updates to existing frameworks like the NIST Cybersecurity Framework and/or MITRE ATLAS. Generative AI’s complexity and vulnerabilities have slowed adoption, underscoring the importance of cross-functional collaboration. Technology introduces sophisticated threats, including advanced phishing and synthetic identities, requiring proactive risk management. Smaller institutions face a significant
disadvantage due to limited access to fraud data, making a centralized data- sharing mechanism important. Additionally, growing reliance on third-party providers raises concerns over data integrity and transparency, emphasizing the need for strong vendor oversight. Lastly, effective governance and skilled human expertise are essential to managing biases, ensuring compliance, and aligning AI with ethical and regulatory standards.
Best Practices for Mitigating AI Cybersecurity Risks
To mitigate the unique cybersecurity risks posed by emerging AI technologies like generative AI, financial institutions must adopt strong, tailored frameworks and foster cross-enterprise collaboration among key stakeholders, including model, technology, legal, and compliance teams. T
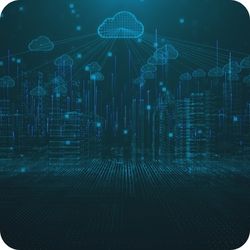
he Treasury recommends embedding AI-specific protocols within enterprise risk management systems, guided by the “three lines of defense” approach to ensure effective oversight across business functions. Institutions should develop customized AI frameworks aligned with standards like the NIST AI RMF while prioritizing collaborative data-sharing mechanisms to support smaller organizations. To strengthen identity and access management, advanced authentication methods and secure-by-design principles must be integrated. Proactive vendor due diligence is critical to evaluating third-party AI capabilities, emphasizing model transparency and data integrity. By embedding cybersecurity considerations from the design phase and enhancing vendor accountability, institutions can better address AI-specific risks and ensure resilient operations.
Challenges and Opportunities in AI for Financial Institutions
Financial institutions face the dual challenge of managing AI’s inherent risks while unlocking its transformative potential to enhance operations, cybersecurity, fraud detection, and system resilience. Addressing these challenges requires bridging the talent gap in AI expertise, harmonizing regulatory approaches, and adopting a common AI lexicon to streamline communication. The integration of AI demands frequent updates, retraining, and substantial investment in workforce training across IT and non-IT roles, such as legal and compliance, to ensure role-specific competence.
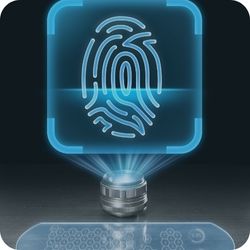
Constrained by limited resources, smaller institutions often rely on third-party providers, highlighting the need for enhanced data sharing and centralized fraud data repositories. Moreover, the lack of explainability in AI models, particularly generative AI, underscores the importance of comprehensive testing and auditing frameworks for black-box solutions. Human oversight, while essential, must be reinforced with skilled reviewers to avoid complacency and ensure informed decision-making. Financial institutions can proactively address these complexities by aligning AI innovations with strategic goals and compliance requirements, paving the way for a secure and efficient future.
Key Insights from the 2025 World Economic Forum Cybersecurity & AI Report
Secure AI adoption allows organizations to innovate while managing risks effectively. As AI becomes more integral to business operations, its misuse or compromise can cause severe impacts. The World Economic Forum’s (WEF) latest report about cybersecurity and AI provide the following key practices that organizations should adopt for the safe integration of AI:
✔️ Risk-Based Approach: Assess and manage AI risks with a structured, risk-focused methodology.
✔️ Cross-Disciplinary Collaboration: Engage diverse teams, including legal, compliance, HR, cybersecurity, ethics, and front-line business units, for comprehensive risk management.
✔️ AI Application Inventory: Maintain an up-to-date registry of AI systems to address “shadow AI” and supply chain vulnerabilities.
✔️ Transition Discipline: Apply adequate controls when moving AI from experimental to operational use, especially in key functions.
✔️ Cybersecurity Investments: Commit to strong cybersecurity measures to protect AI systems and ensure resilience.
✔️ Lifecycle Security: Embed security into AI design (“shift left”) and continually monitor operations (“expand right”) throughout the lifecycle.
✔️ Technical and Process Controls: Combine technical safeguards with human and procedural checks to secure interactions between AI and business processes.
✔️ Information Governance: Enforce strict data governance to ensure AI aligns with organizational policies and data protection standards.
Top leaders must define clear parameters for AI-related decision-making, ensuring alignment with organizational risk tolerance, reward assessments, and broader policies. Key questions should guide leadership in evaluating AI strategies, understanding vulnerabilities, and establishing proper assurance processes. Organizations can strengthen resilience, manage cyber risks, and encourage sustainable innovation with AI by prioritizing these measures.
Key Insights from the 2025 World Economic Forum AI in Financial Services
Per the WEF_Artificial_Intelligence_in_Financial_Services_2025.pdf report, among the most pressing concerns is the potential for AI to amplify misinformation, enabling market manipulation and fraudulent transactions. A particularly alarming threat is generative AI (genAI) misuse to create realistic yet fabricated content, such as deepfakes. This issue has escalated rapidly, with deep-fake related tool trading on dark web forums surging by 223% in early 2024 compared to the previous year.
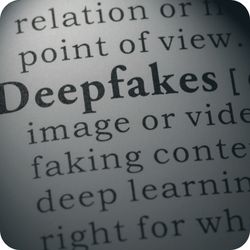
Deepfakes enable lifelike images, videos, and voices with remarkable ease, facilitating sophisticated scams. For example, fraudsters impersonated a chief financial officer and colleagues on a video call, convincing a financial worker to transfer $25 million to a fraudulent account (Scammers siphon $25M from engineering firm Arup via AI deepfake ‘CFO’ | CFO Dive). This incident illustrates how these technologies could destabilize financial markets and harm the global economy.
While these risks are growing, AI is also a powerful ally in combating them. Cutting-edge AI tools are increasingly employed to detect and prevent threats through:
- Authentication Technology: Digital watermarks and metadata embedded during content creation help confirm authenticity, reducing the risk of manipulation.
- Detection Technology: Advanced algorithms scan with vast data sets to locate potential threats, sometimes taking autonomous action to neutralize risks. For instance, AI can identify fake content without needing access to the original or quickly analyze application code to determine if it is malicious.
The dual-use nature of AI underscores the need for financial institutions to remain vigilant. By leveraging AI’s protective capabilities, financial institutions can mitigate the risks associated with misinformation and deepfakes while safeguarding financial markets and maintaining public trust in the global economy.
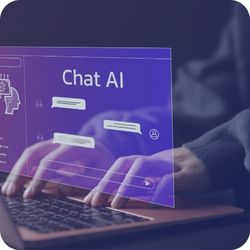
In conclusion, while no significant AI-related cybersecurity incidents have directly impacted banking operations, the potential risks—ranging from data poisoning and adversarial attacks to vulnerabilities introduced through third-party partnerships—underscore the need for a proactive, multi-layered defense strategy. Financial institutions increasingly adopt robust data protection measures, continuous monitoring, and comprehensive governance frameworks integrating security-by-design principles to mitigate these risks. Furthermore, the insights from the FFIEC AI RFI and the WEF reports highlight the importance of cross-disciplinary collaboration, lifecycle risk management, and specialized expertise to navigate the complexities of AI systems. Ultimately, financial institutions can safeguard sensitive data, ensure operational resilience, and foster trust in their AI-driven innovations by embedding these tailored cybersecurity controls and maintaining a vigilant, adaptive posture.
At Mijares Consulting, we recommend financial institutions enhance their workforce development to address the shortage of specialized expertise in AI cybersecurity through several strategies:

Training and Skill Development Programs: Implement comprehensive training programs on AI and cybersecurity. These programs can include workshops, online courses, and certifications that equip employees with the necessary knowledge about AI technologies and the associated cybersecurity risks.

Cross-Disciplinary Collaboration: Create an environment that encourages collaboration between cybersecurity professionals, data scientists, and AI developers. Financial institutions can leverage diverse expertise to better understand and manage AI-related risks by integrating these disciplines.

Partnerships with Educational Institutions: Collaborate with universities and research institutions to create curriculum and internship programs focused on AI and cybersecurity. This partnership can help bridge the gap between academic training and industry needs, ensuring that graduates are better prepared for roles in this field.

Continuous Learning Initiatives: Encourage a culture of continuous learning by providing access to resources such as webinars, industry conferences, and research papers, which can help existing staff stay updated on the latest trends, tools, and techniques in AI and cybersecurity.

Talent Acquisition and Retention Strategies: Develop targeted hiring strategies to attract talent with expertise in AI and cybersecurity. Offering competitive salaries, career advancement opportunities, and a
positive work culture can help retain skilled professionals in these fields.

Mentorship Programs: Establish mentorship programs where experienced cybersecurity professionals can guide new employees which can facilitate knowledge transfer and help junior staff develop their skills more quickly.
Thank you for reading our article on financial institutions’ cybersecurity and artificial intelligence. If you found these insights valuable, please ‘Like’ and ‘Share’ to spread the knowledge within your professional network. Your engagement is crucial in fostering a broader understanding of these important topics.
Don’t forget to “Follow US” for ongoing updates and insights into the intersection of finance and technology. Your involvement is key to building a community at the forefront of industry trends and innovations.
We appreciate your support and look forward to continuing this journey together. Let’s keep the conversation going!
Follow us, stay informed, stay secure, and let’s navigate the risk landscape together.
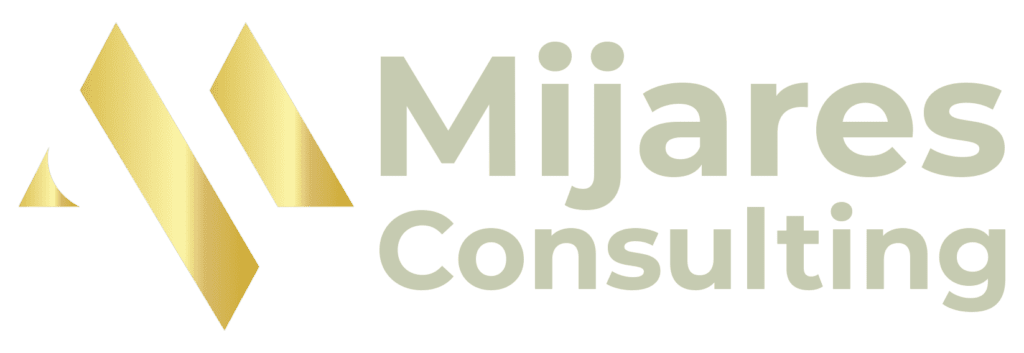